Researchers show how platforms can scrub COVID conspiracies, election lies and other misinformation
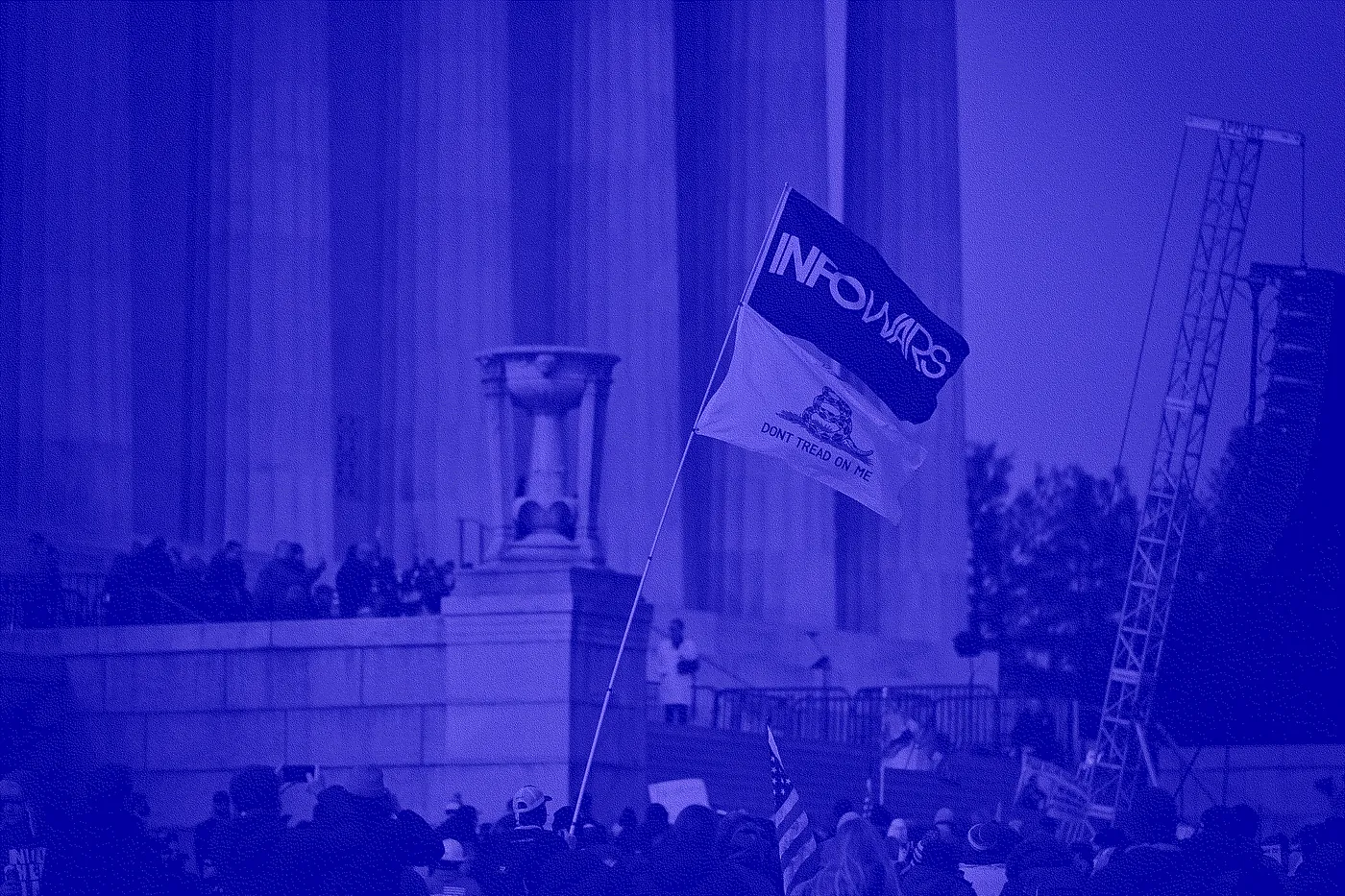
A team of North American researchers is developing a statistics-based technique to weed out falsehoods from social media platforms, with implications for election integrity, cybersecurity and COVID-19.
Social media platforms are hotbeds of misinformation, from pandemic-related falsehoods to election conspiracies. Even though Twitter and Facebook employ fact-checkers and use automated filtering, stamping out deception online is an uphill battle, despite the emergence of so-called “deep learning” tools designed to quickly flag false information by mimicking the human brain.
So, what’s a better way to battle misinformation before it reaches millions of feeds?
Researchers led by American University say they are building an AI model that relies on good old-fashioned statistics rather than the complex neural networks that make up the foundation of deep learning tools.
The advantage of a stats-based approach comes down to trust: While deep-learning tools are fast and efficient, anyone hoping to understand and explain their inner workings is out of luck — a phenomenon known as the “black box” problem.
Statistical models, on the other hand, are less opaque, while their accuracy rates are comparable to deep learning.
They work something like this: Researchers train a machine learning tool to look out for certain characteristics when analyzing a dataset. But they also hand the AI model a statistical roadmap to use for its decision making, so it never becomes a black box.
They “are called transparent models because you can see what’s going on,” said Zois Boukouvalas, an AI researcher at American University in Washington, D.C., and co-author of the study presented last fall at the 24th International Conference on Discovery Science in Halifax, Canada.
“Deep learning models are very accurate,” Boukouvalas told README. “But because they are complex in nature, explainability becomes an issue.”
In machine learning terms, explainability refers to distilling AI decisions into terms a human can understand. For the AU-led research team, which focused on COVID-related misinformation, human subject matter experts categorized a dataset of tweets, flagging a message as misinformation if it promoted political bias, conspiracies, propaganda, anger, or racism.
After further analysis, 17 linguistic characteristics — such as hyperbole, slurs and hate speech, language related to the Trump election campaign or impeachment, and excessive use of punctuation or special characters — were identified as indicative of misinformation. Based on these characteristics, researchers trained the AI model to flag falsehoods using the statistics-based approach. Broadly, tweets featuring more of the characteristics were more likely to be categorized as misleading.
It was imperative that a tweet wasn’t incorrectly flagged, said Nathalie Japkowicz, a computer scientist at AU and a collaborator in the study. But in cases where the AI tool went astray, “the explainability is there to give us some guidance as to why the system [decided] that this tweet should be flagged as misinformation,” she told README.
COVID misinformation has caused considerable damage, with the U.S., along with India, Brazil and Spain, being the top offenders in propagating pandemic-related falsehoods. But even though the AU researchers developed their model to focus on COVID, it can be modified to use with any other kind of misinformation with the right kind of data, such as hate speech or election-related propaganda. “If we had a magic stick to have access to data related to just hate speech, then [we] can throw this data into our system to detect hate speech,” Boukouvalas said.
“Intricately woven” threats
Whether misinformation (which can be unintentional) or disinformation (deliberate falsehoods), the spread of false information can also pose a cybersecurity threat.
The EU DisinfoLab, an NGO that works on researching and raising awareness around misinformation campaigns in Europe, points out that attackers often wield disinformation as part of a wider cyberattack like a “hack and leak” operation. The impacts can cause disruption at a personal, corporate, or even nation-state level.
Misinformation and cybersecurity are two sides of the same coin, said data scientist and diversity strategist Shireen Mitchell, founder of Stop Online Violence against Women and Stop Digital Voter Suppression projects.
“People want them to be separate in a very clean and distinct way…[but] they’re interwoven,” she said. “If someone’s breaching an email system…and then using that data set to distribute a disinformation campaign — that’s intricately woven.”
For the AU-led team, there is still work to be done — including data collection and identifying biases — before a practical application of their model can be developed. The researchers also said they would like to extend the model to analyze images, video and audio apart from text.
Statistics-based models give scientists the opportunity to develop means to keep tabs on what a given tool does and rein it in if needed.